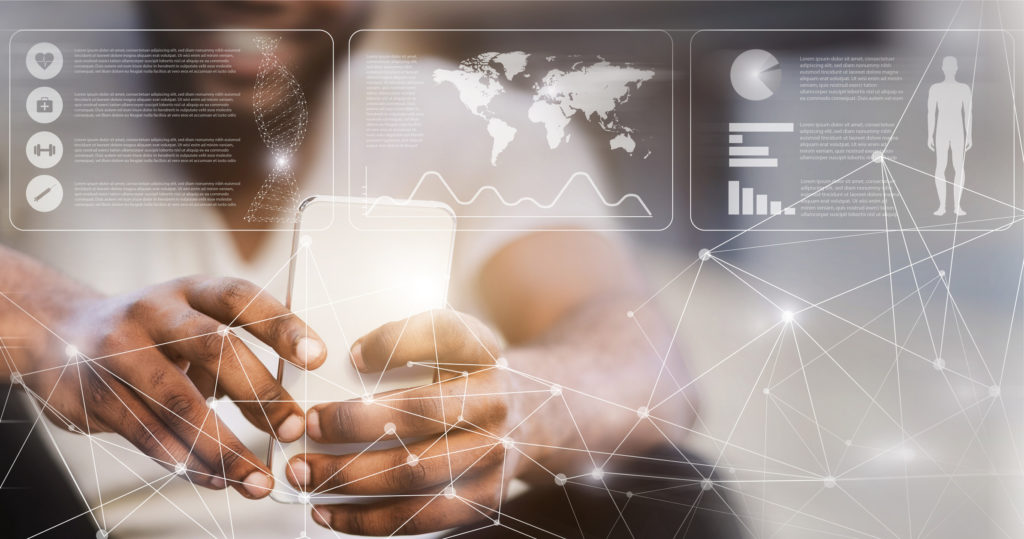
Ddesenvolvimentoimplementadores sãonósing “grandes dados” de celularese remessas para acompanhar o fluxo de refugiados e migrantes.Através dissodados, eles pode modelar o escopo e a escala de um “problema” e fornecer informações em tempo real que permitem que as equipes de elaboração de programas adaptem as intervenções. Pode até fornecer odetalhes quesão necessário para a tomada de decisões de desenvolvimento com base em evidências.
Tudo isso é bom; no entanto, devemos lembre-se dissotais dados geralmente égeradode um subconjunto da população. Por exemplo, cpresságios não estão igualmente representados nessas amostras de dados porque é significativamente menos provável que carreguem telefones celulares, serconectado aa Internetou ativosobre mídias sociais.Portanto, tO uso desses dados exclui automaticamente proporções significativas da população– não só mulheres, mas os idosos, os pobres, certos grupos étnicos – a lista continua. Nesta fase da transformação digital global, big data sofre de representaçãon ou viés amostral e devem ser usados com extrema cautela para informar a tomada de decisões de desenvolvimento.
Grandes dados– particularmentedados passivos, como metadados de mídias sociais e celulares ou registros de detalhes de chamadas–é ouma das principais entradas do modelo ou dados de treinamento paraInteligência artificial(IA) e aprendizado de máquina (AM).
Felizmente, A USAID iniciou um trabalho muito necessáriodiscussão sobre preconceito de gênero em IA e seu efeito nas iniciativas de desenvolvimento. Identificar as formas insidiosas pelas quais o preconceito de género se insinua no ciclo de vida da IA e corrigir esse preconceito é crucial para garantir que a programação futura promova o desenvolvimento inclusivo. Este blog se concentra em obterIA direito de dados subjacente.
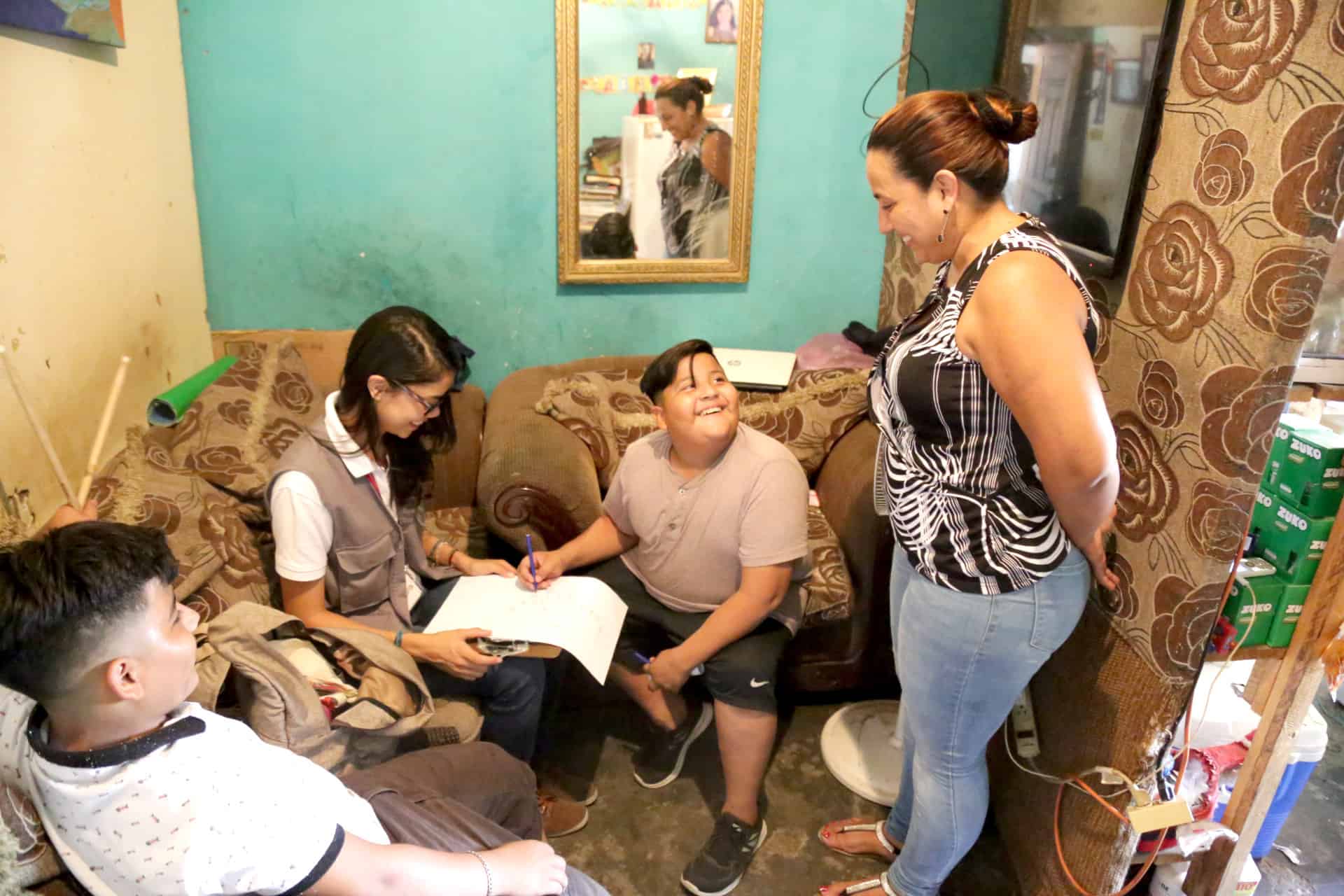
GêneroBias emDesenvolvimento Data
Os actores do desenvolvimento também são produtores de conjuntos de dados através dos esforços de recolha de dados dos projectos.A USAID exige agora que todos os dados do projecto sejam introduzidos na sua Biblioteca de Dados de Desenvolvimento em formatos “legíveis por máquina”. Umpoderia assumir logicamente que isso dados podem ser usados em IA/AM aplicações. Isso seria bom se os dados que vão para os aplicativos de IA estivessem livres de preconceitos. No entanto, os dados de desenvolvimento têm sido atormentados há muito tempo por preconceitos de género.
TO uso de amostras onde os homens estão desproporcionalmente representados é tão prevalente em nosso projeto–conjuntos de dados de nível que muitos de nós nem percebemos. Existem razões práticas para que isso aconteça.
Primeiro, é mais fácil alcançar os homens. Em muitosem desenvolvimentopaíses, as mulheres estão menos presentes nos espaços públicos – seja por causa da sua“pobreza de tempo” (é muito mais provável que eles estejam em casa, realizando tarefas domésticas, cuidando de crianças, os doentes e os idosos) ou devido a normas de gênero. Segundo, as mulheres tendem a ser mais protegidas. As normas sociais muitas vezes restringem os homens–interação feminina e faz com que as famílias hesitem em receber os entrevistadores em casa. Estas normas também tornam mais provável que um homem esteja presente se uma entrevista ocorrer, eé muito mais provável que as mulheres sejam menos abertas em discussões onde os homens estão presentes.Em configurações de grupos maiores, mulheressão mais propensos aseja respeitoso para os homens.
Quer os dados sejam coletados por meios tradicionais ou com base em fontes de big data, o preconceito a favor dos homens nos dados de amostragem é muitas vezes deixado de ladopés incontestado. Justificamos o uso desses dados dizendo a nós mesmosqueisso é o melhor que podemos conseguir. Por isso, oferecemos espaço para que o preconceito de género tenha impacto na forma como interpretamos os dados.Bdados armazenados levam a uma programação que atende às necessidades de alguns (geralmente homens)=e reforçae se propagadesigualdades de género existentes, todosenquantodaring a ilusão de avançar desenvolvimento objetivos.
Abordando o preconceito de gênero no uso de Big Data
Fechando a exclusão digital pode um dia tornar o big data menos vulnerável a preconceitos interpretativos. Enquanto isso, isso énossa responsabilidade de primeiro reconhecer as limitações do uso de big data na captura da realidade local, assim como reflectindo o comportamento das mulheres e de outras pessoas com menor probabilidade de portarem telemóveis ounósing a Internet.
Precisamos então de evitar tomar decisões de programação baseadas apenas em dados que sabemos serem tendenciosos a favor de um subgrupo da população. Estas dificilmente são grandes soluções tecnológicas, mas se implementado com sucesso, minimizariam o potencial de o preconceito de género se infiltrar na forma como utilizamos big data e levaria a decisões de programação mais inclusivas.
Corrigindo paraa representação exagerada dos homensconjuntos de dados devem ser feitos como uma questão de dadosqualidade e melhores práticas, apenas como seria se houvesse outros tipos de distorções nos dados.Tele dadosdefinir precisa ser revisado para garantir queé representativo –nissoistotemnúmero igualé de machos e fêmeas. EUf é distorcido em favor dos homens, então o projeto deve coletar mais dados das mulheres paracorreto pela sub-representação das mulheres.
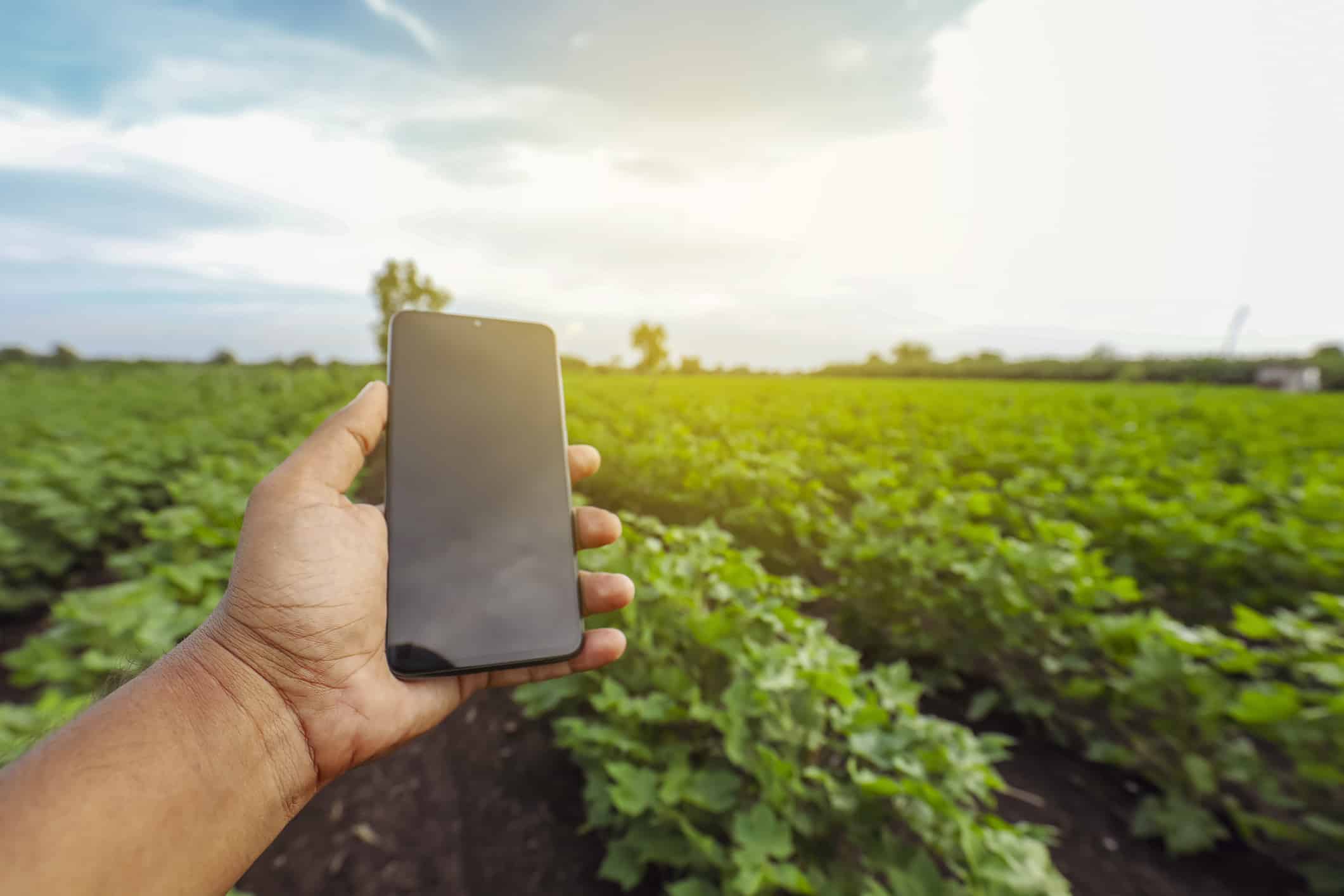
Dando passos positivos
Thoje, nóstêm cada vez mais granularentendimento da extensão da exclusão digital e do acesso digital. Poderíamos aplicar essas informações para desenvolverindicadores estatísticos para permitir que coletores de dados ou editores se ajustem ao viés de amostragem em países específicos.
Se nada mais, nós deveríamosexigirque todos os conjuntos de dados carry etiquetas de advertênciaqueindicar claramente que proporção de homens e mulheres estão representados na amostra. O aviso alertaria aqueles que usam os dados sobreisso élimitaçõese tornar mais difícil para os profissionais do desenvolvimento"inconscientemente"coletar dados de um subconjunto da população e extrapolar as descobertas para toda a comunidade.
Em cenários em que os editores e usuários de dados se conhecem, existe outra maneira simples de expor preconceitos: garantir que todas as partes – aqueles que coletam dados e aqueles que os utilizam – desenvolvam uma compreensão compartilhada dos dados, suas limitações e que conclusões podem ser tiradas dele.
Na minha experiência como Conselheira de Gênero e Inclusão, este exercício tem vários benefícios, o o mais importante deles é interromper“negócios como sempre” e a tendência de confiar em dados tendenciosos. Isto cria espaço para as equipes do projeto reconhecerem a necessidade de desenvolver uma programação que responda às necessidades e aos interesses das comunidades que ficam invisíveis ao usar esses dados.. Sem esse processo, as equipas de projecto muitas vezes não reconhecem a necessidade de considerar as mulheres ou o género – especialmente senão considerá-lo um projeto de “gênero”.
Também recomendamosoprodução colaborativa de um Compêndio sobre preconceito de gênero na IA para o desenvolvimento paraexpor onde e como o preconceito de gênero manifesta e discoé o potencial negativoresultadosna programação de desenvolvimentosetores. Esse cum seja útil avisar discussão eelevaçãoconsciência depreconceito de género nos dados para a tomada de decisões sobre o desenvolvimento.
Nós nãotertodas as respostas, mas sabemos que quanto maisnósdesempacotare analyzedfontes de dados ume entradas no modelo AI/MLé, construir processos que nos forçam aexplorar coletivamente como os interpretamos e os usamos com cuidado para informar nossa tomada de decisão, quanto maisequitativonossoos resultados serão.
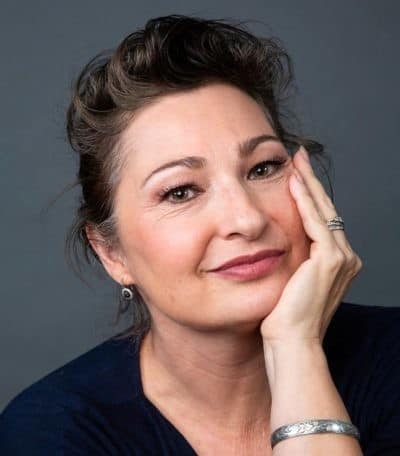
Rebecca Sewall é o Conselheiro Sênior, Gênero e Inclusão. Membros do Laboratório de Desenvolvimento Criativo, promove e canaliza novas estratégias para enfrentar os desafios do desenvolvimento através da ciência, tecnologia e mídia, contribuiu para este blog.